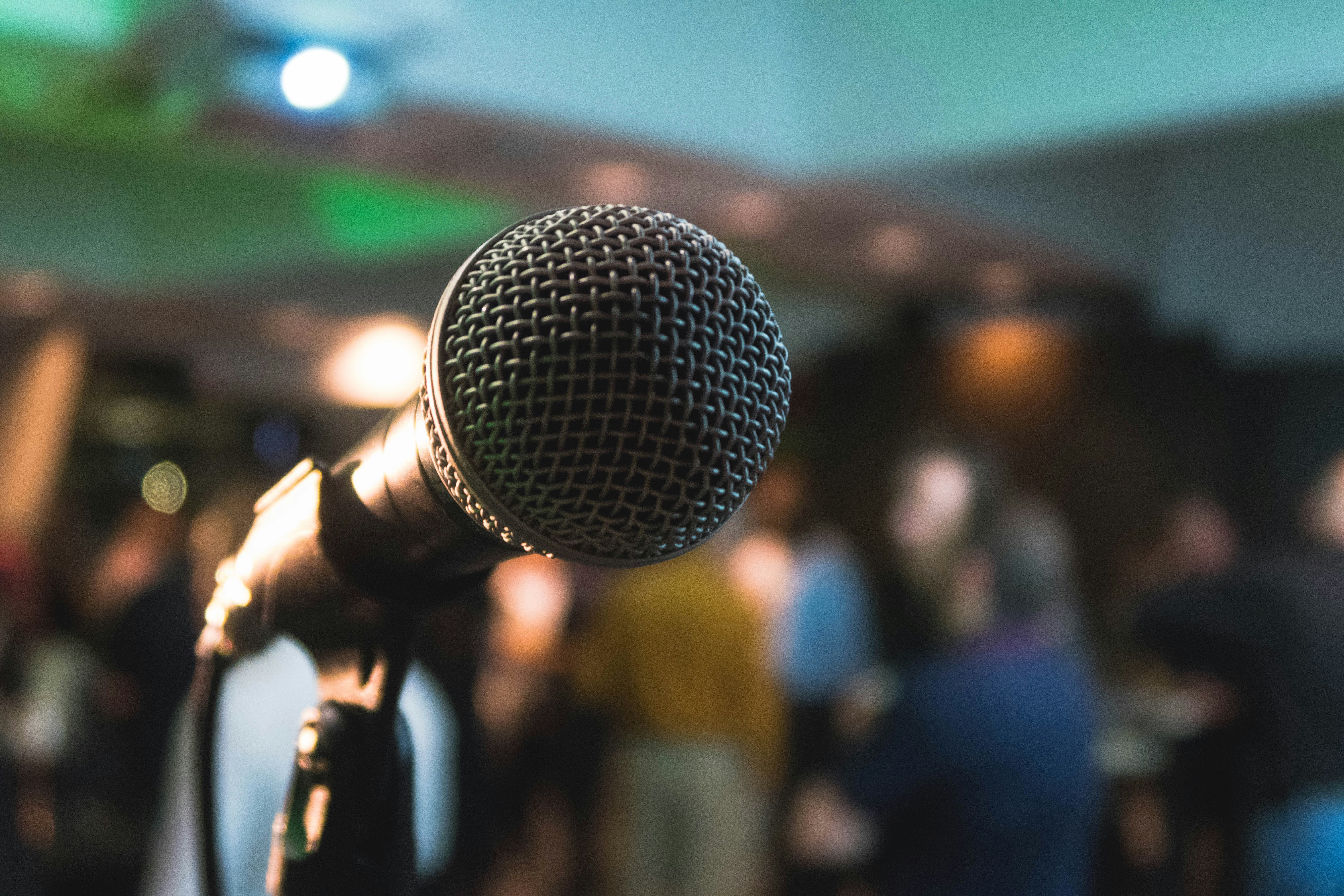
How to Present AI Models to Non-Technical Audiences: A Public Speaking Guide for Job Seekers
In today’s competitive job market, AI professionals are expected to do more than just build brilliant algorithms—they must also explain them clearly to stakeholders who may have no technical background. Whether you're applying for a role as a machine learning engineer, data scientist, or AI consultant, your ability to articulate complex models in simple terms is fast becoming one of the most valued soft skills in interviews and on the job. This guide will help you master the art of public speaking for AI roles, offering tips on structuring presentations, designing effective slides, and using storytelling to make your work resonate with any audience.